By Mathieu Boudreau
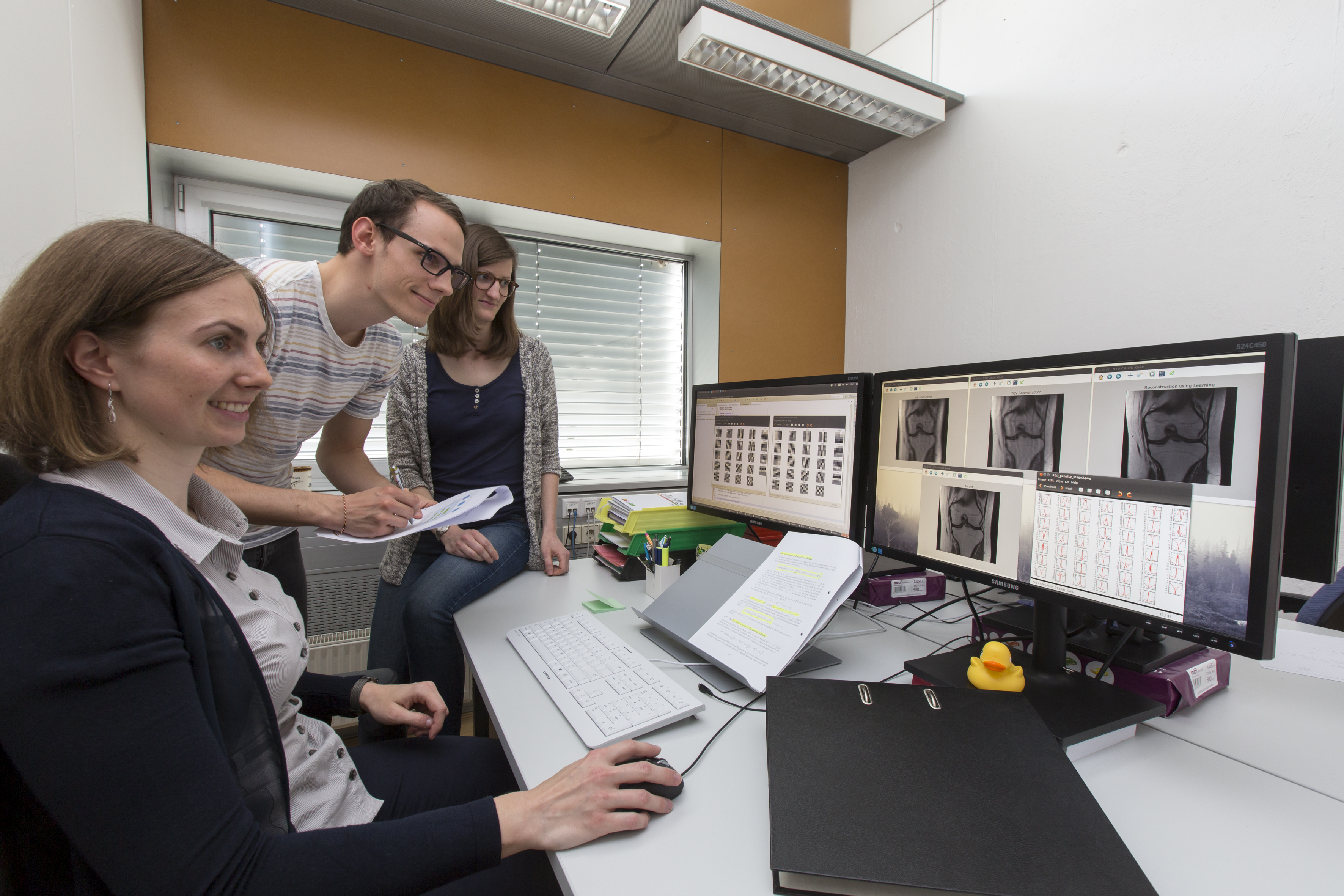
This first June 2018 Editor’s Pick features Kerstin Hammernik and Florian Knoll, researchers at Graz University of Technology in Austria and New York University School of Medicine (NYU), respectively. Their paper presents a novel approach to MR image reconstruction in which a generalized compressed sensing framework, formulated as a variational model, is combined with deep learning. Tested on a musculoskeletal disease patient population, their reconstructions preserved the natural appearance of MR images and worked well with pathologies that were not included in the training dataset. We met them to find out more about this project and the people behind it.
MRMH: First of all, could you fill us in on your backgrounds?
Kerstin: I did my Bachelor’s and Master’s degrees in Biomedical Engineering. For my Master’s, I focused on image processing, specifically 3D registration and segmentation of dental data and vertebrae using CT images. I’m currently doing a PhD in Computer Science which is supervised by Prof. Thomas Pock. It wasn’t until after I started my PhD that I began working with Florian, in the field of medical image reconstruction.
Florian: I actually come from Graz, where Kerstin is currently doing her PhD and I did my Master’s and PhD there as well. I became interested in machine learning, the focus of my Master’s, some time ago, before it became the hot topic it is today. I then did my PhD in MR imaging. After that, about five years ago, I moved to NYU, where I’m mainly responsible for the development of new methods in data acquisition and reconstruction, and their translation into clinical practice.
MRMH: Could you give us a brief overview of your paper?
Kerstin: The main idea was to come up with a generalized compressed sensing framework, formulated as a variational model. Our reconstruction model has a data term, which allows us to fit the collected k-space data and transform the image with an operator into k-space, and also a regularization term, which imposes prior knowledge on the image. We then combined the variational models with deep learning to learn the parameters of the regularization term and data term in an unrolled gradient scheme, and applied this combination to reconstruct accelerated MRI data. We did experiments on clinical knee data, and looked at how the network performed for different contrasts, orientations and SNRs. We also performed a reader study to evaluate the difference between the compressed sensing methods we tested and our deep learning approach.
Florian: It perhaps helps to understand what first prompted us to do this work. When talking with clinical radiologists, we always encountered resistance when presenting them with images using our compressed sensing reconstructions: they would complain that the images looked a little unnatural and say they weren’t confident making a diagnosis based on them. We discovered, over the course of this research, that handcrafted models (like total variation) overly simplify the image content, resulting in this unnatural cartoonish appearance. Our approach allows us to use much more complex image models that can describe the character of our images better. When the method we presented in this work is used as a regularizer, it results in images that are more acceptable to the human eye.
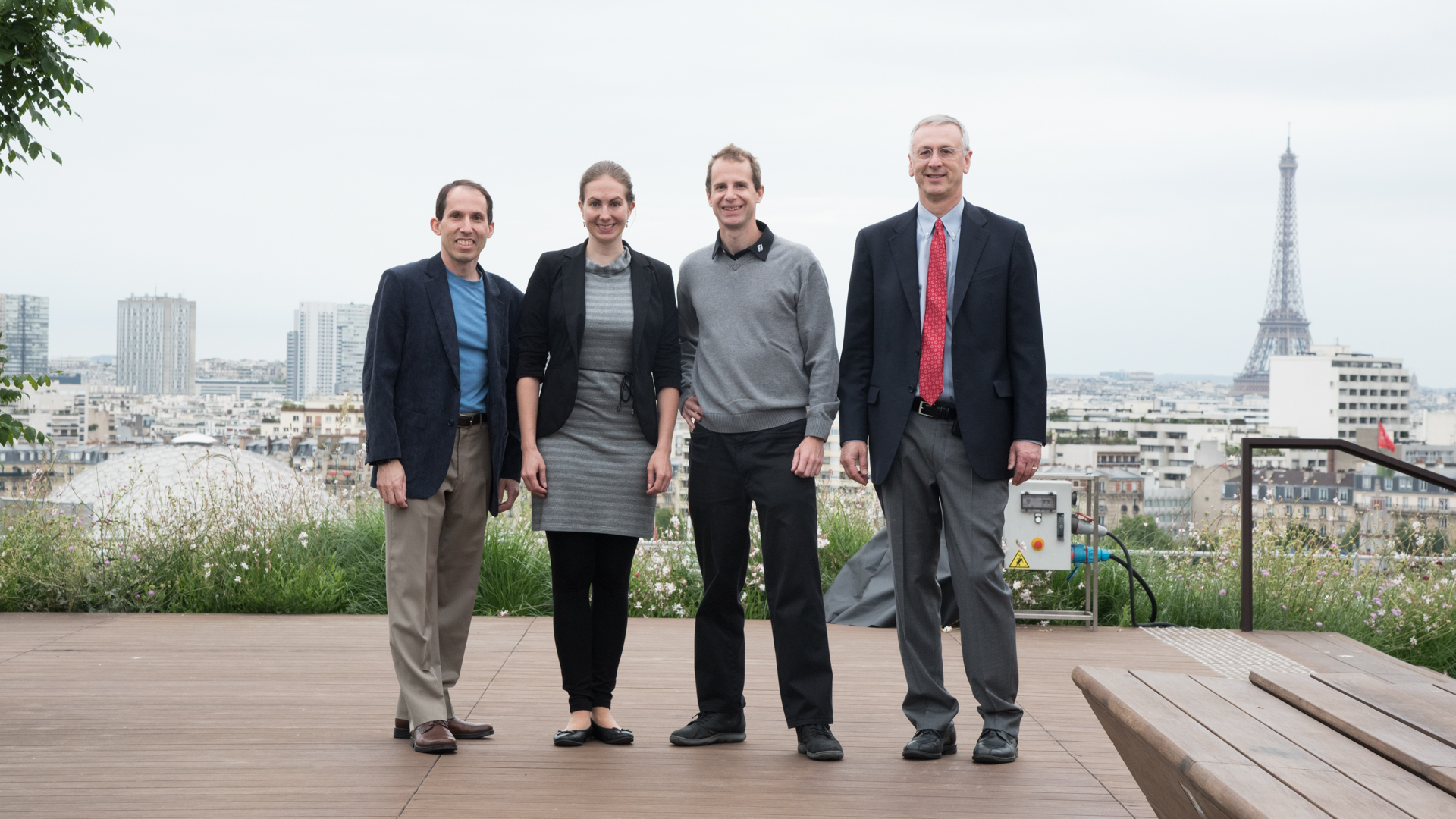
MRMH: Were you surprised by any of the results of this study?
Kerstin: When things work, you’re always surprised! [laughs]
MRMH: Where would you like to see this work going from here?
Kerstin: It would be very interesting to establish whether this really is a feasible method for clinical exams – whether it could be applied to a clinical workflow.
Florian: Clinical validation is obviously really important. There are a lot of unanswered questions, and people are always skeptical when they hear the words machine learning. We need to show, in a reasonably sized patient population, that we can produce images that have complete diagnostic value.
MRMH: Are there any other particularly exciting aspects you’d like to highlight?
Kerstin: This method is a tool of exploration and discovery that may be applied to what we already know from compressed sensing. We have shown with our filter kernels and learned functions that we actually get filters and functions that have already been studied and reported in the literature. The next step is to look at whether the number of parameters can be reduced by imposing some structure on the models, and if so how this can be done. This is an important research direction for me.
Florian: At the moment, we are still considering image reconstruction as a step that is isolated from the data acquisition and the diagnostic pipeline. But later on, once we have established that images can be reconstructed in this way, we can look at whether it is possible to go back and change the way we acquire the data, and whether this can help to improve the diagnostic interpretation of the images and the post-processing as well. Furthermore, with the substantial improvements that are constantly being made in computing power and data science, could some of the traditional constraints on our imaging hardware be relaxed by incorporating novel calibration and correction procedures in the reconstruction procedure? All these are certainly exciting questions and ambitious objectives.
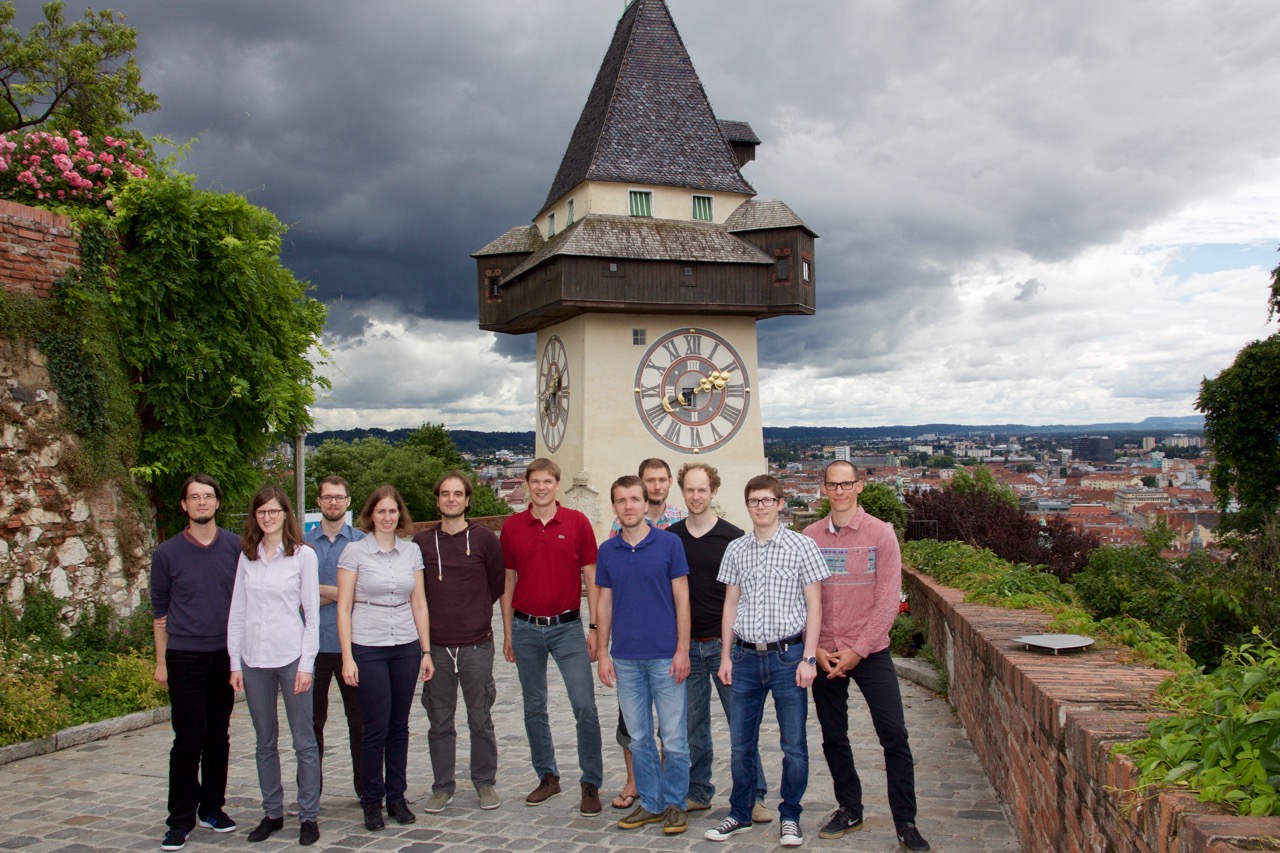
MRMH: What do you enjoy doing when you’re off duty?
Kerstin: It’s important for me to really switch off and relax in my spare time. I love dancing – styles like salsa, bachata, kizomba and zouk. I also love to be outdoors in the mountains and do ski touring, hiking or mountain biking.
Florian: I mainly do sports stuff too – marathons, and martial arts.
MRMH: Have you run in any of the major marathons?
Florian: Of the big city marathons, I ran New York and Chicago. For my next challenge I am considering doing a trail race. That would make an interesting change!
Code and sample data from this work is available on GitHub: https://github.com/VLOGroup/mri-variationalnetwork