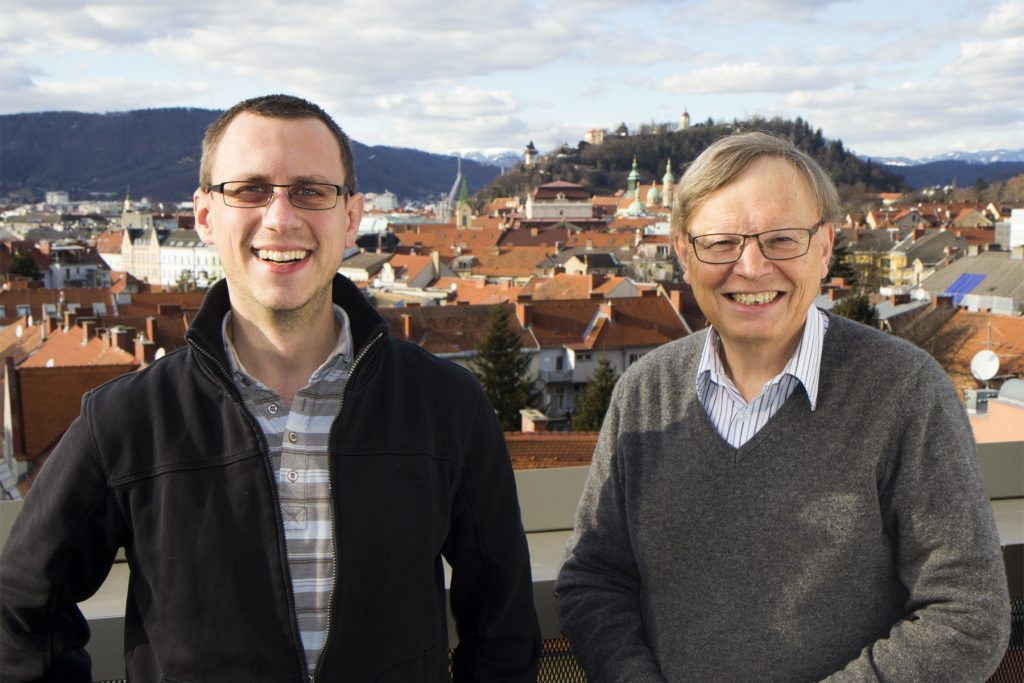
By Mathieu Boudreau
This Editor’s Pick interview is with Andreas Lesch and Rudolf Stollberger, researchers at Graz University of Technology in Austria. Their paper presenting a variational modeling algorithm for reconstructing B1+ maps from highly accelerated Bloch-Siegert data demonstrated that even acceleration factors of up to 100 can produce good quality B1+ maps, allowing quantitative maps to be acquired in the space of a single breath-hold. We recently spoke with them to find out more.
MRMH: Andreas and Rudolf, could you introduce yourselves and tell us a little bit about your backgrounds?
Andreas: I completed my bachelor’s degree in biomedical engineering in 2011 here in Graz, and got my master’s degree in the same field in 2014. For my master’s I focused mainly on medical imaging, and my thesis was on T1/T2 mapping using bSSFP with slice profile correction.
Rudolf: I started out in electrical engineering, also doing some courses in biomedical engineering. This was around the time that the first MRI scanners were appearing. I then went to Zurich to study MRI, before coming back to Graz, where I worked in the clinic for about 20 years in the medical physics and radiology department. Now I’m at the Graz University of Technology (Technische Universität Graz), as a professor of medical engineering.
MRMH: Before we get into the details of your work, could you explain the concept of variational modeling?
Andreas: Variational modeling is based on a minimization procedure of a cost function that includes a data fidelity term, where you measure the similarity of your reconstructed image to the measured data, and regularization terms, which model some behaviors of the underlying image.
Rudolf: In principle, you try to find an unknown function that represents the image you are looking for. In variational modeling, we have more flexibility than in traditional fitting since we do not define an explicit function. There are a number of solutions depending on encoding, sampling and the noise of the given problem, and therefore it’s necessary to add some properties of the solution, which is done by regularization.
MRMH: Please give us a brief overview of your work.
Andreas: In this paper we described a mathematical formulation that we developed in order to regularize the underlying B1+ field, and developed an image reconstruction algorithm to produce B1+ maps out of highly undersampled data. Furthermore, we evaluated the influence of different undersampling patterns, how to choose the right regularization parameters, and applications to different anatomical regions.
Rudolf: Basically, we were extending the Bloch-Siegert method to variational modeling.
MRMH: Did any of your results surprise you?
Andreas: We were surprised that our approach worked so well. Even with very low amounts of data, we could still achieve good estimates of the B1+ field, which I hadn’t expected. I was also surprised that the optimal strategy was to use the same undersampling patterns for both acquisitions.
Rudolf: In dynamic imaging, it’s typical to change the sampling pattern from frame to frame. We assumed this would be the case for this application, too, but our results showed that it is not.
Andreas: It could be that our algorithm can reduce artifacts more properly if they appear in the same way in both images.
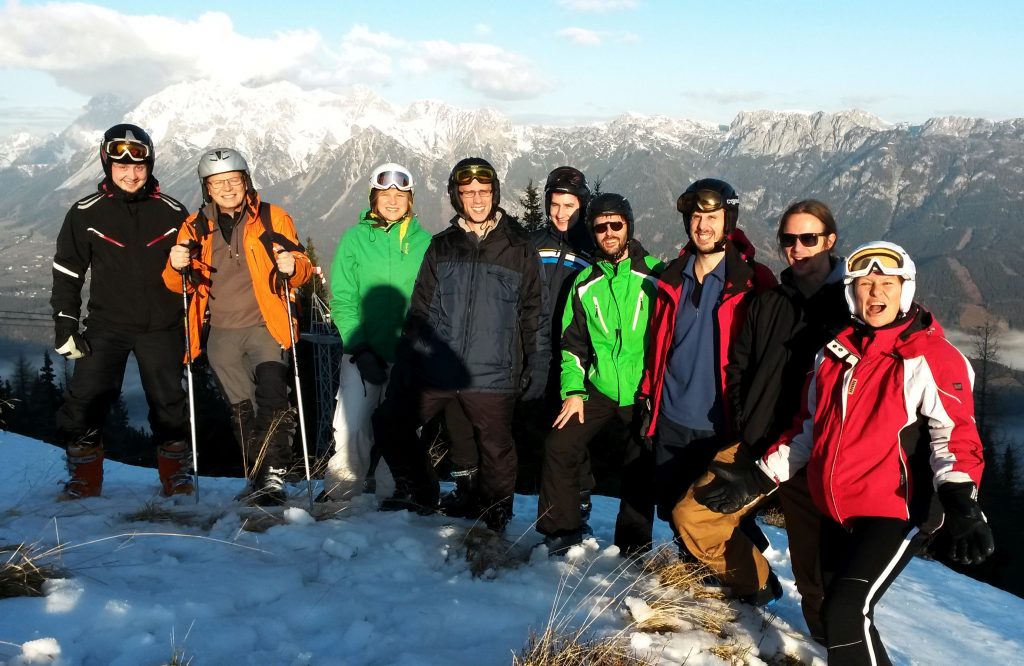
MRMH: You reported an accelerating factor of up to 100 for this technique and application – do you think this can be improved even further, or are we near the information limit?
Andreas: [laughs] In terms of k-space lines, I don’t think much more acceleration would be possible, because we only have a very small amount of data. But we are currently working on combining this method with an EPI readout in order to improve the speed of the measurement without reducing the quantity of information acquired.
Rudolf: We should really discuss whether it is even acceptable to call it an “acceleration” of a factor of 100, given that we found that value for a specific image matrix size. Theoretically, if we acquired a higher resolution image with the same field of view, then we might be able to use a higher acceleration factor to reconstruct the profile of the B1+ function. So it may not be reasonable to talk of an acceleration in the same way as we do for other techniques used to reconstruct conventional MR images.
MRMH: How does this research fit in with your broader research goals?
Andreas: We are using it in some quantitative MRI projects that I am partially involved in. I also did some work on fat-water separation, another situation in which there is an important background field, the B0 field.
Rudolf: For me, this is a special topic because my PhD thesis was on B1+ mapping [smiles]. It was on the double angle technique; I first presented it in an abstract in 1988, and later on I published it in an MRM paper. Here, the Bloch-Siegert method has one advantage over older, more common techniques, namely that it also produces the absolute value of B1+. This is important for certain applications, such as CEST (Chemical Exchange Saturation Transfer) imaging, which we are also exploring at the moment.
MRMH: Why did you choose to make your code open source?
Andreas: Because we wanted to share our results with other researchers, in the hope that they might further develop them or to use them for their own research. Had we kept the code for ourselves, this would only have created more barriers in the usability of the work we presented in the paper.
MRMH: What do you enjoy doing when you’re not in the lab?
Andreas: For me, it’s very important to do activities that relax my brain! I really enjoy hiking and climbing in the mountains. I also like to travel a lot, all round the world.
Rudolf: Well, I grew up in the Austrian mountains, so I’m also an outdoor guy. I like hiking, mountaineering, skiing and biking in the summer. I also like gardening at home, where I grow tomatoes and other Mediterranean plants and herbs.