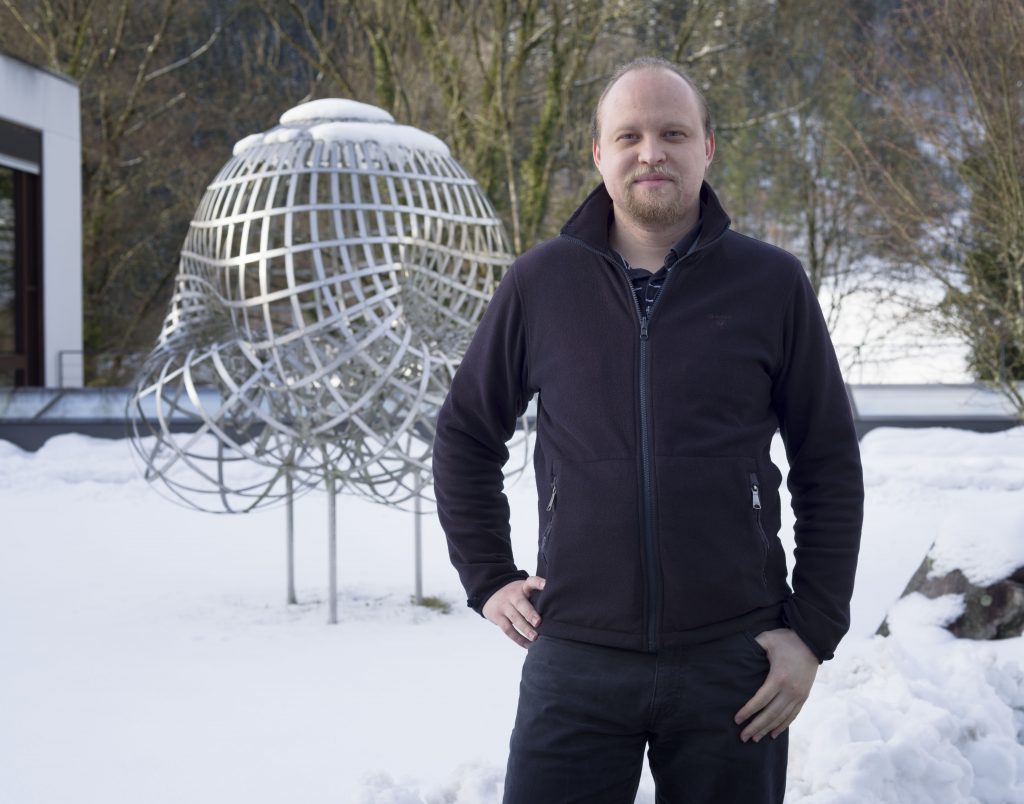
By Agah Karakuzu
MR image reconstruction has become a magnet for deep learning and cardiac imaging is definitely playing a part in this! For our second Editor’s Pick of the month, we interviewed Dr Andreas Hauptmann and Prof. Vivek Muthurangu about their paper on real-time artifact suppression for accelerating real-time cardiac exams using deep learning. It is worth noting that their method can reconstruct images superior in quality to those obtained with compressed sensing, yet without sacrificing acquisition speed.
MRMH: Can you tell us a bit about yourselves? What sparked your interest in MRI research?
Vivek: I’ve been involved in MRI since 2002. I am a clinician, but I also work in MRI physics. From a clinical point of view, my main interest is congenital heart disease both in children and adults, so I am very interested in fast imaging. As you can imagine, overcoming problems like breath holding and motion is really important if you are working with children.
Andreas: I am relatively new to MRI. I got my PhD in applied mathematics from the University of Helsinki. My research dealt with inverse problems and focused in particular on medical imaging. About two years ago I started my postdoc at University College London in the Centre for Medical Image Computing, where our research group was already collaborating with Vivek’s group. This led us to discuss how we might combine our expertise, and we came up with the idea of applying deep learning to cardiac imaging.
MRMH: Can you explain your paper in a few sentences? What was the driving motivation for it?
Andreas: When we first discussed the possibility of using deep learning for reconstruction, we realized that we already have a large dataset of magnitude images from the past 10 to 20 years that might serve as the ground truth for a supervised training project. Basically, we created retrospectively undersampled data, obtained the corresponding undersampled reconstructions, and trained a network to remove noise and artifacts, basically a sort of denoising network. As the first tests with the simulated data worked out really well, we proceeded to use this trained network on prospectively undersampled data. We were quite happy with the results from this reconstruction, too. For us, it was not necessary to beat compressed sensing (CS) in terms of reconstruction quality, but really to obtain clinically useful reconstructions with a considerable speed-up. As a clinician, image quality is not enough without information quality. I am happy to sacrifice a bit of image quality, as long as the information is the same. Speed is important, though, and that was the real push here.
Vivek: I would like to talk about clinical motivations. My group is involved in developing non-Cartesian real-time imaging, leveraging various kinds of reconstruction, ranging from parallel imaging to k-t SENSE. More recently we have started using CS and have been getting pretty good image quality. The big problem is reconstruction time, even when the reconstruction is performed on a GPU. For example, a recent spiral SSFP real-time sequence that we developed can take up to 10 seconds per slice to reconstruct, depending on the hardware. That may not sound like a long time, but if you are attempting to complete the whole scan in 10 minutes, reconstruction time becomes an issue. So, we decided to take a different approach by leveraging the large amount of image data that we already have.
MRMH: The model you trained with retrospectively undersampled data worked like a dream in reconstruction during new in vivo scans. Did this outcome exceed your expectations?
Andreas: Of course our approach didn’t work right from the beginning on the prospective data. The first tests on the scanner gave decent results, but they were far from perfect. The most important aspect in order to get the trained network working for prospective acquisitions was consistency between simulations and the data acquired from the scanner. Once we managed to get the undersampling artifacts in the simulations to resemble, sufficiently closely, those from actual prospective data, the network indeed worked like a dream, and yes, we were really surprised to see how well it worked in the end!
Vivek: Personally, I was extremely surprised to obtain such good results for both retrospective and prospective data. An important aspect of our method is that the synthetic data must be created in such a way that it closely resembles real-time data that is going to be acquired in real life. This requires a little bit of work when you start with retrospectively gated Cartesian Cine MRI data and are trying to create pseudo real-time radial acquisitions. You have to do this properly and if you get it right, the reconstruction works extremely well. Furthermore, this reconstruction outperformed CS in terms of image quality. This is a really important point, as I and my clinician colleagues often find that CS data has an odd, cartoon-like image quality. One of my colleagues calls this the “disneyfication” of cardiac MRI. We don’t see that in the machine learning reconstruction.
MRMH: The choice of sampling pattern seems a critical aspect, and continuously rotating tiny golden angle sampling (tGAro)t comes out top in this regard. How are you going to make use of this information in your future studies?
Andreas: Yes, the sampling pattern is really crucial for denoising temporal reconstructions. For the network to perform properly, we need the aliasing artifacts to be noise-like structures in time. That means our network primarily denoises in the temporal dimension, rather than learning how to reproduce structures from the training data. In fact, even if we change the target completely it manages to create good reconstructions without reproducing features learned from the training on hearts only. For our approach to work properly, in future studies, we will really need to use efficient sampling patterns creating artifacts that are incoherent in time.
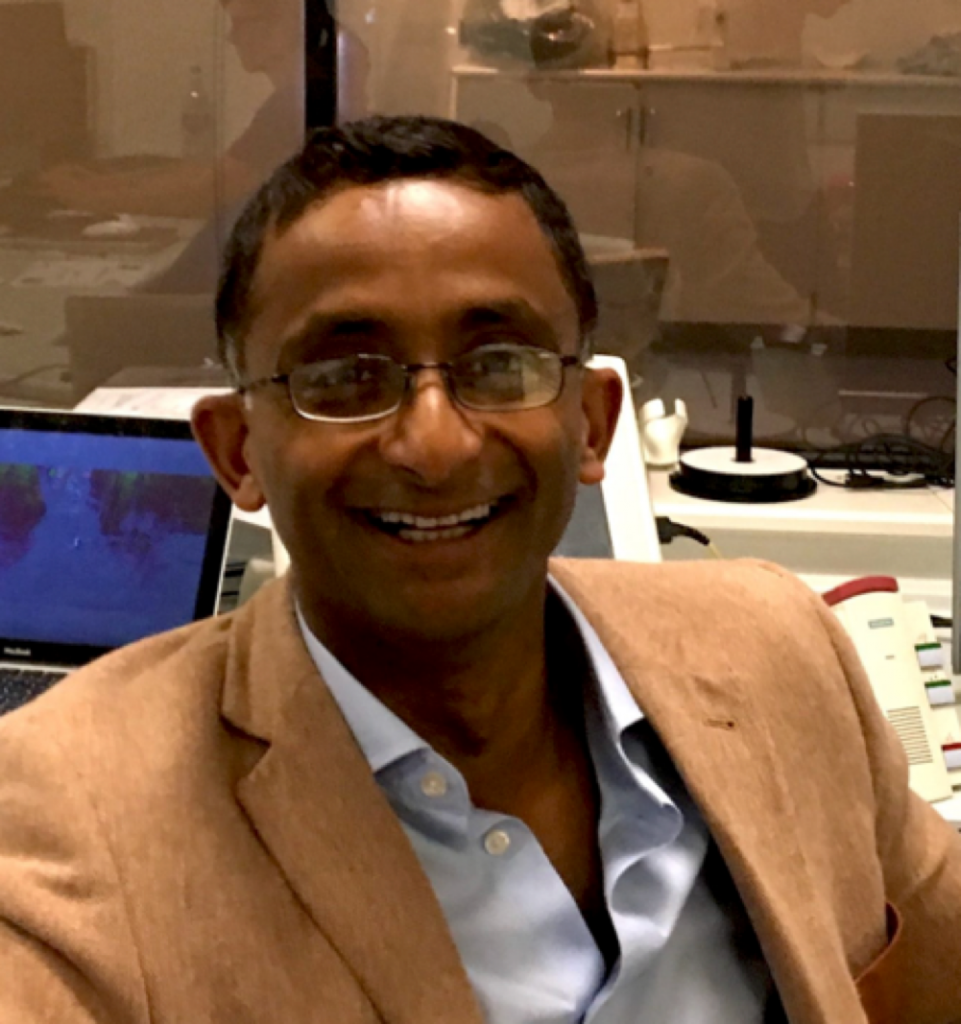
Vivek: Aliases have to look like noise in our approach. The whole idea is to reformulate reconstruction as a denoising problem. For example, we did some testing with spiral acquisitions and found that the results were not as good as with radial acquisitions. This is because aliases are less incoherent and don’t have a noise-like appearance. A lot of work has been done by the CS community to produce these noise-like artifacts with different types of sampling. I think we can build on these findings for machine learning reconstruction, too.
MRMH: Bias in training data is not desirable for diagnostic efficacy. How do you see initiatives such as ISMRM raw data format (ISMRM-RD) clearing the way for consistency?
Vivek: We have run a few tests on the effect of bias in reconstruction. The whole network was trained on images from patients who have two ventricles. We prospectively scanned one patient who had one ventricle, and it still worked beautifully. Initially, bias was something we were worried about, but the way we implemented our machine learning reconstruction seems to overcome this problem. As for ISMRM-RD, I think it is a fantastic resource for machine learning reconstructions. However, for our implementations, we need magnitude data and there needs to be a parallel standard for this type of data.
MRMH: In this ever-changing artificial intelligence (AI) landscape, can you imagine AI-powered reconstructions as end products able to fit clinical reality?
Andreas: Our driving incentive for the study was to see whether our approach was clinically applicable. We have previously encountered some limits with CS reconstructions, especially in terms of reconstruction speed. Given the competitive results of our study, I see a big opportunity here for the clinical end-use of this method and machine learning in general.
Vivek: We shouldn’t develop techniques if we can’t use them clinically. For these machine learning reconstructions to have clinical uptake, people have to believe in them. This means that you can’t just validate new techniques in 40 patients and convince people that they work. You have to demonstrate this in hundreds of patients from multiple sites. People have bigger concerns about machine learning, as it is considered a sort of a black box. I think machine learning is a technique that holds clinical promise, but we need to be transparent in the way it is developed.