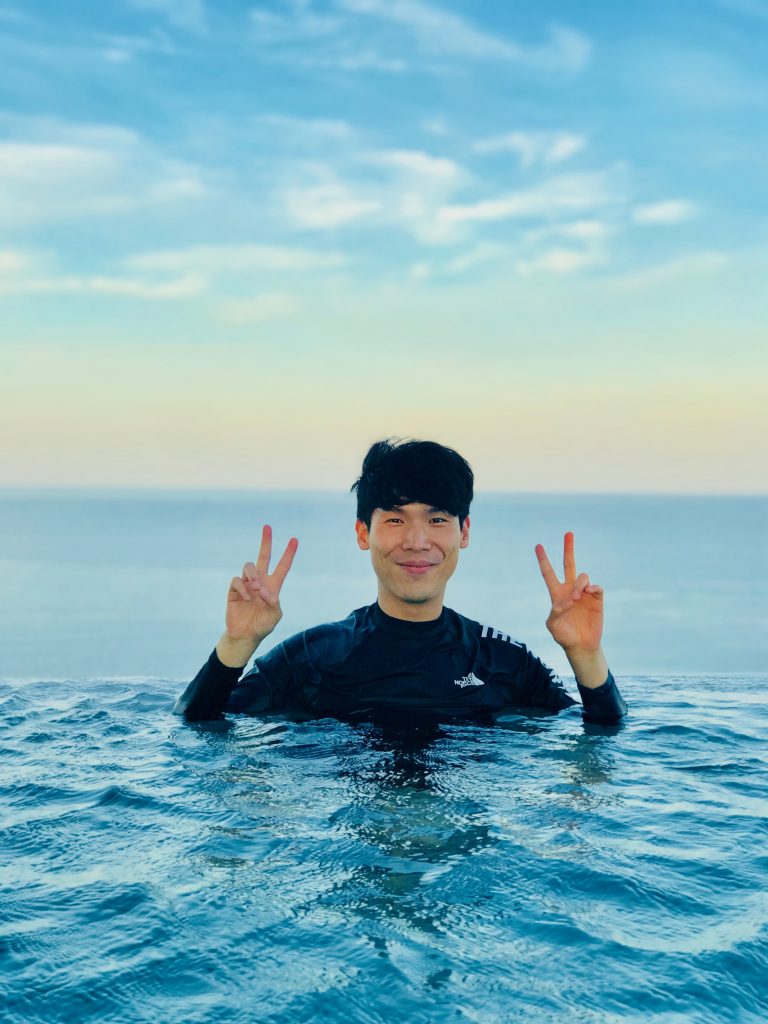
By Mathieu Boudreau
The first September 2018 Editor’s Pick is from Yoseob Han and Jong Chul Ye, researchers at Korea Advanced Institute of Science & Technology (KAIST) in Daejeon, Republic of Korea. Their paper presents a deep learning approach that allows streaking artifacts to be removed from undersampled MR images acquired with a radial k-space acquisition pattern. They used a deep learning network pre-trained on a large CT dataset and then used a small MRI dataset to adapt it for their application. They demonstrated that this process (domain adaptation) performed better than other reconstruction techniques (e.g. compressed sensing), and has the added benefit of a much shorter image reconstruction time (post-training). We recently spoke with Yoseob and Jong about their project.
MRMH: First of all, we’d like to know something about you and your background.
Yoseob: My name is Yoseob Han and I am a PhD candidate at KAIST in Korea. During my Masters I worked on CT reconstruction techniques using compressed sensing, and my current interest is image reconstruction using deep learning, for both CT and MRI applications.
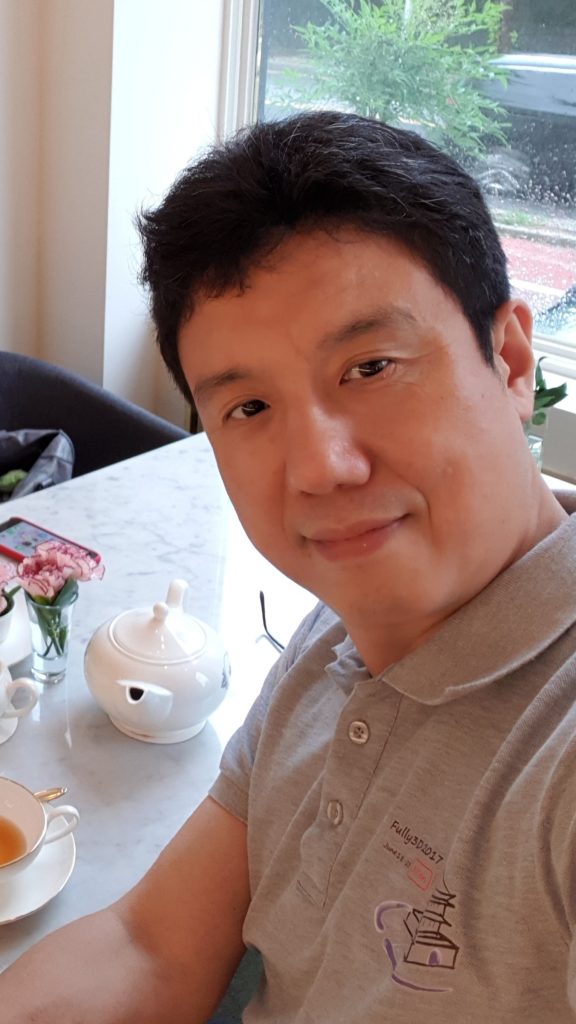
Jong: I am Professor Jong Chul Ye, Yoeseob’s PhD advisor. Our research group has been working in the MRI field for nearly 14 years, but we don’t only do research in this field – we are interested in image reconstruction problems encountered in various biomedical applications, such as CT, PET, ultrasound and optical techniques. We focused on compressed sensing for many years, and developed some well-known techniques in the MRI field such as the k-t FOCUSS algorithm and ALOHA. However, in the past two years we have turned our attention to deep learning approaches applied in biomedical imaging reconstruction, starting with CT, and then moved on to our present work with MRI.
MRMH: Could you give us a brief overview of your paper?
Yoseob: The goal of the proposed method was to use deep learning to remove the streaking artifacts from MR images corrupted as a result of accelerated acquisition performed using a radial k-space pattern. The challenge to overcome was the lack of sufficient MRI data to train the deep learning network adequately. To resolve this issue, we set out to pre-train the deep learning model with a large CT dataset, which has a similar radial acquisition pattern, and then perform domain adaptation by fine tuning the CT-trained model using the limited MRI data available for the particular application. On using this technique, we discovered that our network outperformed some existing compressed sensing methods with much shorter image reconstruction times.
Jong: In fact, the takeaway message from this paper is that it is not the end of the world if you don’t have enough data to train a neural network for your application. You simply need to have enough data to train it in another domain with a similar acquisition pattern. And for this purpose, you don’t only have to use real data, such as CT images; you can also use simulated data, provided the acquisition trajectory is similar.
MRMH: Could you clarify what you mean by “domain adaptation”?
Yoseob: If a neural network is pre-trained with a specific dataset (in our case, CT), then we say that the network is biased for this domain (referred to as the source domain). Accordingly, our network cannot reconstruct the corrupted MR images well because it has not learned the characteristics of MRI data (that is, of the target domain), and is biased for the source domain (CT). But because the two domains contain similar information, deriving from shared characteristics (e.g. radial acquisition pattern), then we can adapt the source domain to the target domain by fine tuning it with, in this case, MRI data.
Jong: Yoseob himself is actually an example of domain adaptation [laughs], because he started his career learning about CT and deep learning for CT reconstruction, and then I asked him to adapt his knowledge of reconstructing CT images using deep learning to another application: reconstructing accelerated MRI images with radial trajectories. We didn’t need to retrain him from scratch [chuckles].
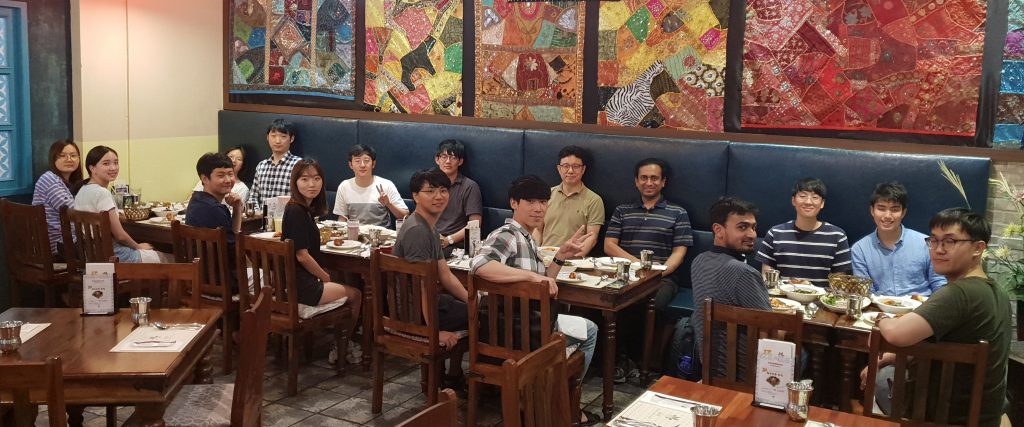
MRMH: What are your main “takeaways” from this study?
Jong: Many people may initially be concerned about not having enough data to apply deep learning approaches in their MR reconstructions. But one take-home message is that if you use domain adaptation or transfer learning techniques, you can still do a decent reconstruction even with limited data, provided you have another well-trained model in another domain.
MRMH: Where do you see this work heading? Have you thought of any exciting ways of using or extending it?
Jong: I think the main challenge with model-based regularization techniques like compressed sensing is their very long reconstruction times, but as we have seen, this is now being overcome by deep learning, an approach that can easily be adapted to learn from big data. And furthermore, even though the training takes a long time, real-time reconstruction is much faster with deep learning, and that’s actually all that practitioners are concerned about. So, this is really where the image reconstruction field is heading, and we are proud that this paper may contribute to progress in this direction.
MRMH: What do you enjoy doing when you’re not in the lab?
Yoseob: I recently went to Seoul with my girlfriend to visit the Han River. It was great, and we ate some very delicious food [laughs].
Jong: Yeah… he’s a young guy [laughs]. I myself usually enjoy playing with my kids. Daejon is a science town, and we have a lot of national labs based here. It’s a good area to educate children, and I enjoy life here. But if you want to enjoy more fun stuff, like this young guy [laughs], then you can easily get to Seoul, which is just an hour away by train.
The pre-trained deep learning networks for this work is available online.