Nicole Seiberlich is the Elmer Lincoln Lindseth Associate Professor in the Department of Biomedical Engineering at Case Western Reserve University, and her research focuses on rapid quantitative imaging. Nicole delivered the 2017 NIBIB New Horizons Lecture at the ISMRM annual meeting in Honolulu Hawaii, and we used this opportunity to ask her a couple of questions about her life and her career.
Adrienne Campbell-Washburn (ACW): How did you get started in MRI?
Nicole Seiberlich (NS): When I was an undergrad, I studied chemistry and we talked about nuclear magnetic resonance (NMR) for characterization of compounds. You’d have to infer from the spectrum exactly what you were looking at, and I thought that link was really exciting. So, I joined an NMR lab and then, for my senior year project, I worked in an electron paramagnetic resonance (EPR) lab. I really liked it. When I finished my bachelor’s, I went on to be a financial services consultant for two years, knowing that I was going to come back eventually to academia and do my PhD. Through a series of weird coincidences, I ended up in Germany, started looking for a lab in Wurzburg, and the lab that I randomly fell into was an MRI lab. This was Peter Jakob’s lab where Mark Griswold was working at the time. That’s how I got into this field.
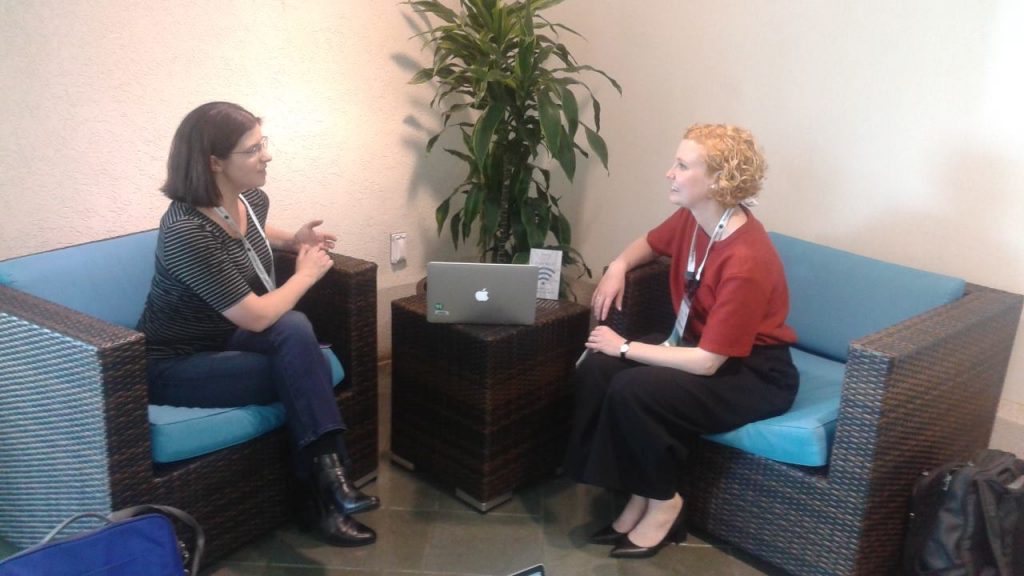
ACW: A lot of your work is focused on fast imaging; what drives you to make imaging faster and faster?
NS: I wish I had a more personal story to tell, but, for me, it’s just interesting signal processing. I think it’s exciting to be one of the first people to see a new kind of image, like, we did a really fast real-time non-Cartesian parallel imaging reconstruction and as soon as the movies popped up in MATLAB, I was like “no one’s ever seen that before”. I think that is a really exciting moment. So, it’s not necessarily the clinical application, per se, but being able to pull out this new information that you know must be in your data in order to visualize something new. I think that’s awesome.
ACW: And the title of your NIBIB lecture was “unleashing the power of numbers” – could you explain why you think numbers are so important?
NS: Quantitative imaging is the future. I mean, if you look at other imaging modalities, the signal intensity of the pixels means something. And when we look at our images, it’s all relative. That seems to be a shame because we have all of these different tissue properties that we’re sensitive to in our signal. It’s OK to have them all in one qualitative image, but we can use signal processing to extract that specific information from our data. I think that everything is coming together at the right time to make quantitative imaging possible and take it into a clinical setting.
ACW: So, we’d be missing an opportunity if we didn’t do it now?
NS: Exactly. Now is the time. We have really fast readouts, different types of sequences, sparsity based reconstructions and techniques to pull apart the multidimensional nature of the data. These ideas are not new, but we’re able to use them, especially with advanced computing, in a way that wasn’t there before.
ACW: Fingerprinting has become a mainstream topic here at the ISMRM meeting – what do you think it is about that technique that’s so appealing?
NS: I think it’s a new way of thinking about information coming out of the scanner. It is not just images. I think with fingerprinting there was just this break where we said we can use our physics in a different way to design a sequence that forces signals to look totally different, and then we can figure out what the tissues are, as long as they look different enough. I think also people are very skeptical of fingerprinting for exactly that reason; that it’s changing the way you’re looking at the information that is coming out of your scanner. One of the cool things about fingerprinting is anything that the scanner is sensitive to, including system properties, we can also potentially map, and if you can model the system differences into the reconstruction that may allow us to come up with completely reproducible quantitative maps. I think that’s a really powerful idea. We like this idea of open source, which is what research should be, and as more people are trying our new variants, it is really exciting for us.
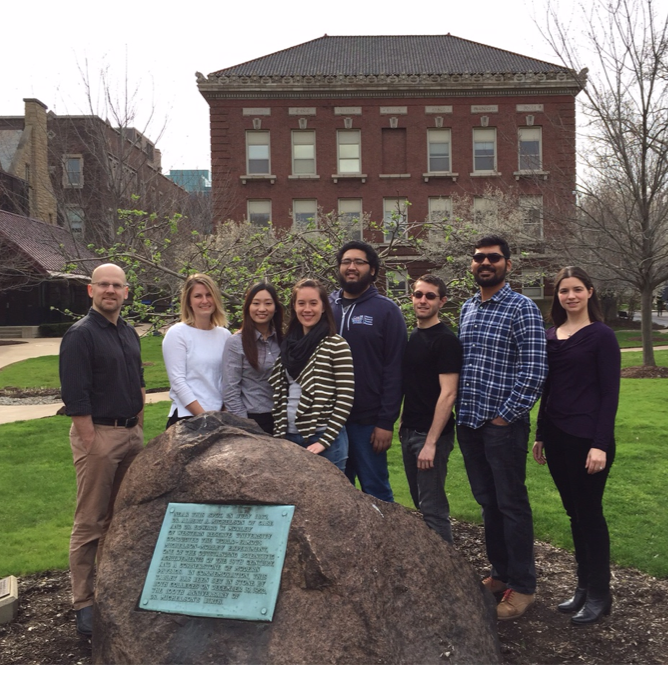
ACW: Can you tell us about your efforts to standardize fingerprinting?
NS: We’re working with the National Institute for Standards and Technology (NIST) and we are using the NIST/ISMRM MRI system phantoms at 5 different locations that are all performing fingerprinting measurements in order to determine how reproducible the results are. So far, they are surprisingly reproducible across all sites. It’s slow going because we want to make sure that we are deploying the same sequence and we are using a standardized phantom. I think there’s the natural desire, once you get something that’s working, to say “OK good, we’re going to use it on 5000 people”, and we’re really trying not to do that.
ACW: From a practical perspective, what do you think are the most important steps in taking something from the research realm to the clinical realm?
NS: There’s the technical development step, which, as a physicist, is the part that I like best. You have an idea, you sit down in Matlab, you scan a phantom, you scan 10 people and you publish your paper. I think a lot of things die there, because the next step is by far the hardest step, which is somehow convincing some clinical scientist to deploy this new, weird thing, that doesn’t have online reconstruction and it fails the first 100 times you run it on a patient; and to convince them to help you figure out how to tweak it. I think often basic scientists and clinical scientists speak different languages, even when they are really trying to connect. As much as I’d love to just hand something off, you just can’t do that if you really want your technology to succeed.
ACW: It’s important to really integrate it into their workflow and make it easy for them.
NS: Absolutely. We are so painfully aware of how difficult that can be. We’ve just made a quantum leap by transitioning to using Gadgetron (http://gadgetron.github.io/). We want to be able to quickly and flexibly implement our reconstruction algorithms on a platform that we can then share with other people. Plus there is the capability to connect it up to your scanner so that the data goes right out and the reconstructed images come back in. I have an amazing student who has taken some of the reconstruction algorithms that we have in Matlab and she has not only put them in Gadgetron, but also made them fast in Gadgeton with all the GPU capabilities. Every day she is like “oh, now I can do real-time 3D cardiac imaging” and I’m like “woah, we couldn’t do that yesterday”.
ACW: Do you have any particular “Eureka moments” in your career where you’ve been working on a problem and the solution finally came to you?
NS: I think the biggest Eureka moment came to me when I had been working on the radial GRAPPA approach. It wasn’t working very well and I was presenting to the lab an idea that I wanted to try. Jeff Duerk said something that was just so obvious, and I don’t think that he really understood the full implications of what he had said, but it was this moment where I was like “oh my gosh… you’re right!”. The problem was coming up with calibration data for non-Cartesian data sets. Basically, what Jeff said is “If your high acceleration factor is going to allow you to get very high frame rates, it’s OK if you spend a little bit of extra time with the patient in the scanner. It’s the temporal resolution which is actually what you need, so if you can spend a minute collecting calibration data and then you can fly when it comes to actually collecting data, then it’s worth it.” That idea of disconnecting those 2 processes was just a brilliant moment. Within probably 3 days we were doing real time cardiac imaging with non-Cartesian parallel imaging. It was crazy.
ACW: Switching gears a little bit, I wanted to ask you what it’s like being on the ISMRM board of trustees and how you got there.
NS: I got there because Clare Tempany and the Nominating Committee decided to put me on the ballot, which was really an honor! I figured I would run a couple of times and, maybe, eventually, I’d be able to make it. So, I was really shocked when I found out I was elected to the board. I think it’s good to have different kinds of people representing the society on the board. It’s really interesting to see the inside machinery of the ISMRM and how decisions are made and to help shift the directions in which it might be going.
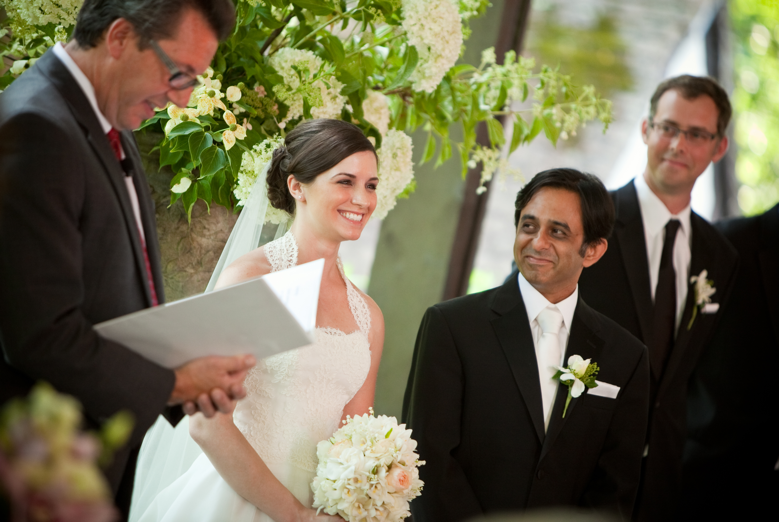
ACW: I was wondering if you had any advice for junior scientists who are just starting out on their career?
NS: I think science, in general, is hard. Things don’t work the first few times you try them. It’s research. That’s why you’re researching it, because it doesn’t work very well and as soon as it starts working, then you move on to another problem. I think that scientists get used to their research not working, but I think the same persistence is needed when it comes to the professional aspects of science like submitting papers, submitting grants, practicing talks and even, you know, putting your name in for the board. You just have to keep at it and don’t take it personally when it doesn’t work out. Even when it feels like “oh, you rejected my paper, does that mean you don’t like me?”. No, it just means that you need to do some work on the paper. It can be harsh sometimes, but keep at it and don’t take it personally.
ACW: Where do you think the field will be in 10 years?
NS: 10 years? I think we’ll be completely quantitative. Dan Sodickson has this idea of the continuous comprehensive scan, and I like this idea of sticking someone in the scanner and just collecting data, then after the fact extracting all the information you need whether it’s tissue properties or motion or 3D anatomy. It’s this idea of collecting information rather than collecting images. We’re no longer going to be constrained by Fourier reconstructions and breath holds and all the things we do artificially to make that one perfect image. I think we’ll be able to do MRI guided interventions a lot better and we’ll be able to scan completely in real-time and visualize in real-time, potentially using some of the newer visualization technology like full 3D holographic representation of what you’re trying to look at. Maybe that sounds a little bit crazy, but I think that all the elements are there right now, in fact we might even be further than that in 10 years, maybe it’s not ambitious enough.
ACW: There’s been a lot of machine learning presented this year, how do you think that will fit in?
NS: I think that will play a big role. I think a lot more will be, and should be, automated. Especially when our images are quantitative it will be easier to say “the signal in this area is high and the signal here is really low and that’s intermediate and all of that together equals prostate cancer”. People are saying radiology as a field is not going to be here, and I don’t think that’s necessarily true; we’ll still need radiologists in order to put all of that data together to actually make a diagnosis.
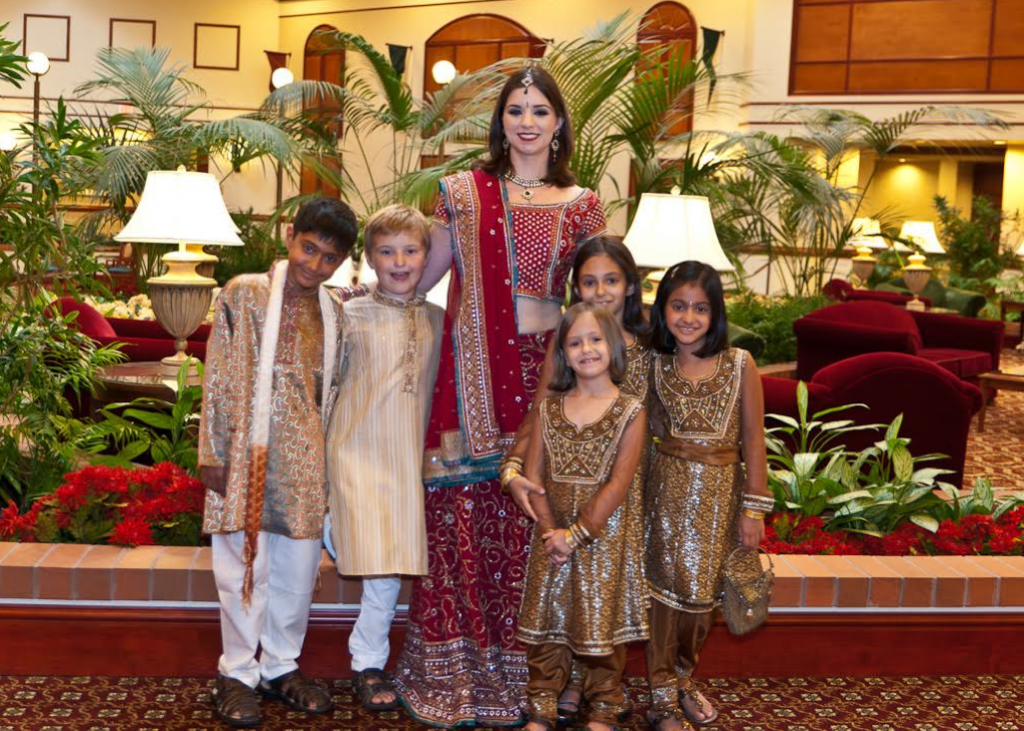
ACW: If you weren’t doing research, what would you be doing?
NS: I like to solve problems and I think we have a lot of problems in the US and the world right now. I’m not saying that the people who are going at those problems aren’t extremely intelligent, but I think being trained as a scientist sometimes you can think a little bit differently about a problem and that is actually the most powerful thing that we learn how to do. Not just to do the step-by-step scientific process, but to step outside of the box and say: “why are we doing this, maybe we should do something totally different?”. I think that kind of thought process might be used well in making policy decisions.
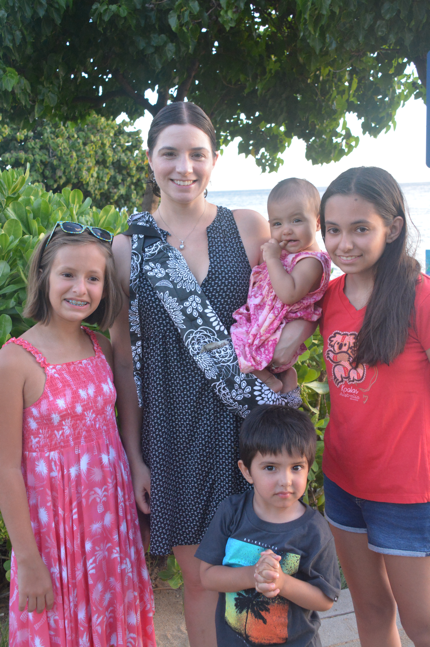
ACW: Who are you outside of the lab?
NS: Well, I don’t teach anymore but I’m a trained yoga instructor, so I try to do yoga when I can. I have 4 children, a 17 year old, a 13 year old, a 3 year old and a 1 year old. They’re awesome! We have a really busy house right now, but I think that it works because Vikas (my husband who is also in MR) and I, we’re pretty good at understanding the time constraints of the other person.
ACW: Anything else you want to mention?
NS: I also do want to say that my lab is the greatest lab in the universe. The way it works at Case Western is that several scientists, including Mark [Griswold], Vikas [Gulani] and I have different labs that are under the umbrella of the big MRI lab. The big MRI lab is fabulous, but my lab is just amazing. I can’t believe the things that they come up with and the way that they work together. I think that they are just crazily creative people and really fun to be around and I’m really honored to have them in my life. I love my lab!