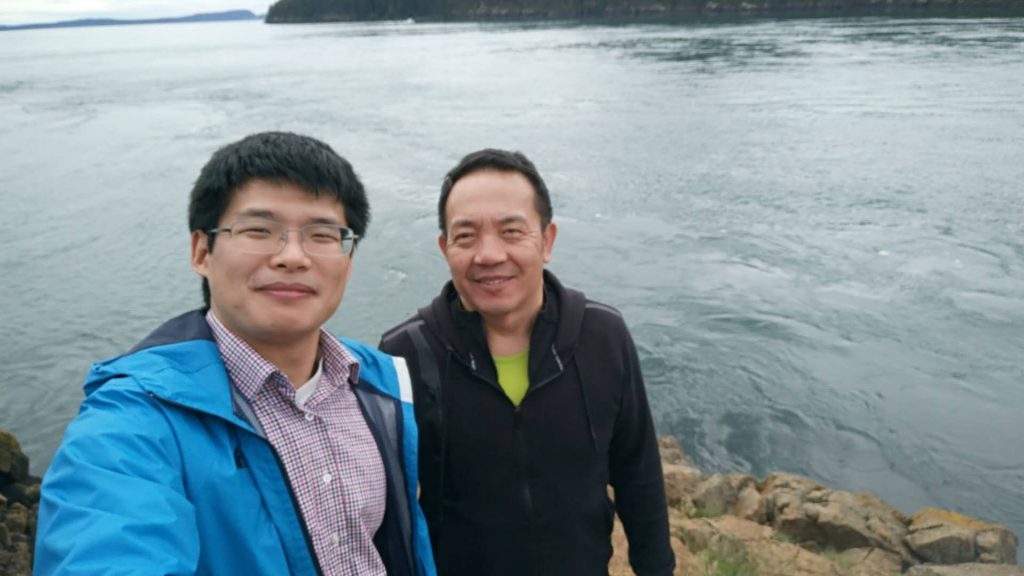
By Giulia Ginami
The second Editor’s Pick for June 2018 is a paper entitled “Development of a quantitative intracranial vascular features extraction tool on 3D MRA using semiautomated open-curve active contour vessel tracking” by Li Chen and Chun Yuan of the University of Washington in Seattle. I caught up with Li and Chun during the ISMRM conference in Paris, where we took a break from the meeting’s incredibly packed and stimulating program to talk about their work.
MRMH: To begin with, could you tell us how you came to work in MRI, and in Seattle?
Li: My introduction to this field was an MRI course in Shanghai, which quickly taught me that MRI is not only about equations, but can provide quantitative information that can significantly impact on people’s lives. The instructor on that course went on to become my mentor, and under her guidance I began focusing on vascular image processing. I then reached out to Chun — the possibility of working with him sounded like a great match! — and indeed my transition from undergraduate to graduate studies went very smoothly. And so, here I am!
Chun: Well, I started working in MRI in 1984, way before Li was even born! My mentor was Prof. Dennis Parker, who was working in the field of vascular imaging. My PhD thesis focused on MR flow. I ended up in Seattle as I wanted to live on the West Coast. California was not an option, and I like to be close to the mountains. The University of Washington has an excellent cardiovascular biology program and is also strong in imaging, and I am therefore very happy to work here.
MRMH: Could you give us a brief summary of your paper?
Li: Our paper describes an intracranial artery feature extraction tool. The intracranial arterial system has a very tortuous geometry with significant individual variations. We set out with the idea of generating, starting from an MRA image, a reconstruction of the arterial tree, in order to then extract morphometry and intensity information for quantifying any physiological or pathophysiological changes that occur.
Chun: The crucial thing to understand here is that information about the vasculature, in terms of its distribution, tortuosity and length, can tell us about the state of the blood flow in the brain at any given time. Indeed, our target isn’t just stenosis: our aim is to put together a complete description of the cerebral vasculature, in the hope that we can then see how it changes over time.
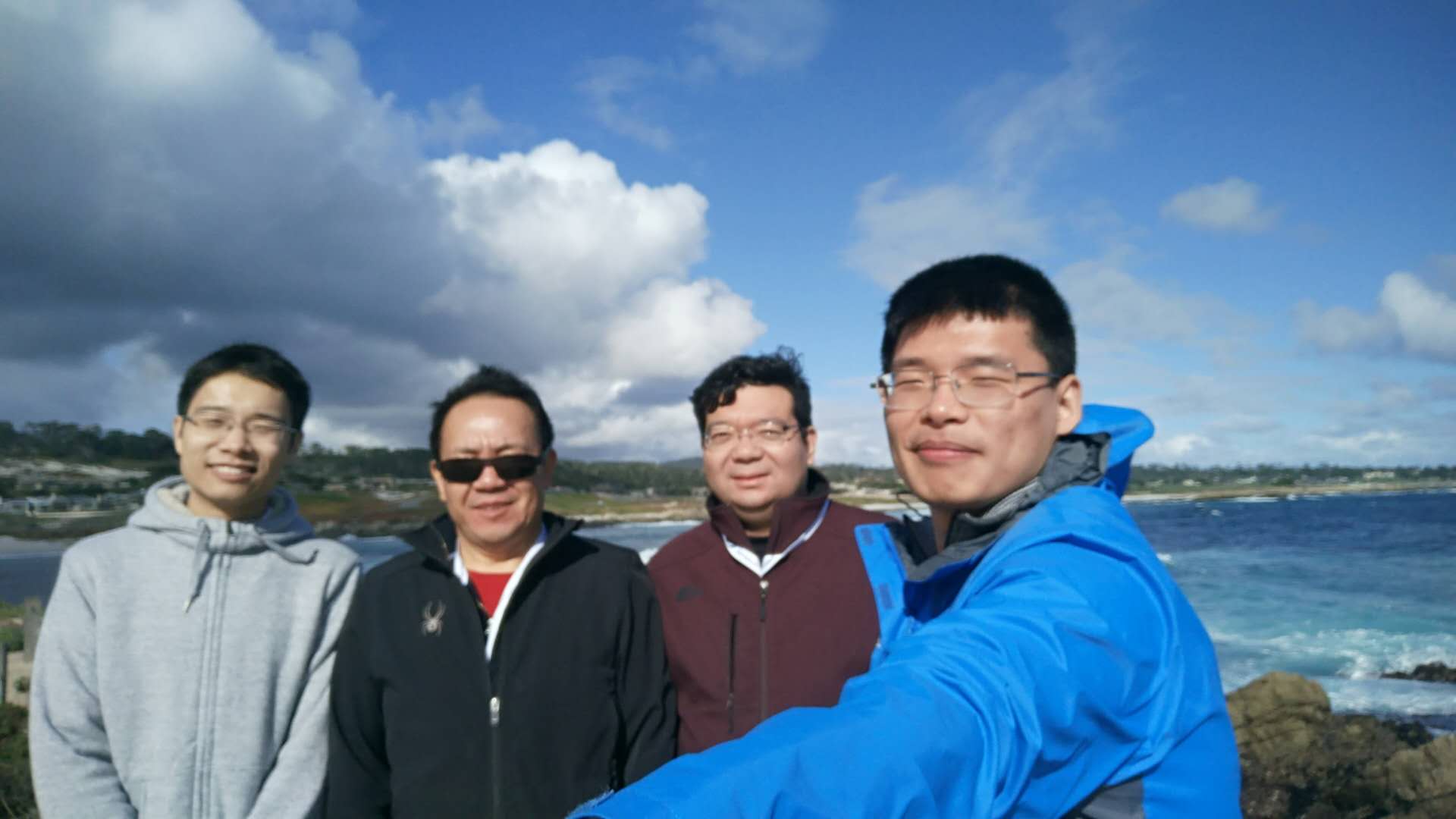
MRMH: How does your approach differ from other feature extraction tools?
Li: We believe the features extracted from our tool are comprehensive and accurate. The tool is comprehensive in the sense that it allows us to extract all arteries, even the distal ones with radii of less than one pixel. Furthermore, in addition to tracing the vessels, we can also divide and label them into 24 types, which is more than most previous tools can do. With this comprehensive labelling process, we can extract not only global but also regional vascular features of interest. The accuracy of the tool derives from the fact that the arteries, once automatically traced, can easily be edited by humans, thereby ensuring the quality of the artery reconstruction results.
Chun: I don’t think it would be fair to say that our method is only about accuracy, as we are certainly working on different aspects. As I already said, we don’t typically focus on stenosis. I would say we aim to obtain an accurate description of the vascular map, and particularly of sub-regional bases. And it’s also important to keep things simple. After all, if tools take days to process, this may hinder the broader clinical implementation of these techniques.
MRMH: What can you tell us about the manual editing part of your algorithm? Do you think it could be extended and made fully automated in the future?
Li: At the present stage, human interaction remains crucial in order to obtain an accurate map. It is very hard to design a fully automated yet accurate feature extraction tool. We feel that, for now, the manual editing part actually confers added value on our tool. In the future, however, combining our approach with the machine learning technique may help to reduce the importance of the manual editing part.
Chun: In this era of artificial intelligence, I believe that deep learning algorithms may significantly improve the performances of this feature extraction tool. 3D time-of-flight images are routinely acquired in many centers. The information that we are trying to extract is not yet used clinically, but it is hugely important from a research perspective.
MRMH: There are many applications that would benefit from the vascular feature extraction approach you are proposing. Do you think it could be applied in other human organs, too?
Li: The approach exploits contrast between foreground and background, therefore it could potentially be extended to many applications. We are currently applying it to peripheral arteries. Cardiac or abdominal vasculature are other possible options. Here in Paris, I have been asked several times whether it might be applied to retinal images or vessels of mice, which are really fascinating possibilities. But with different applications, the distribution of the vessels and the intensity of the background may change, of course, and therefore optimization steps may be needed.
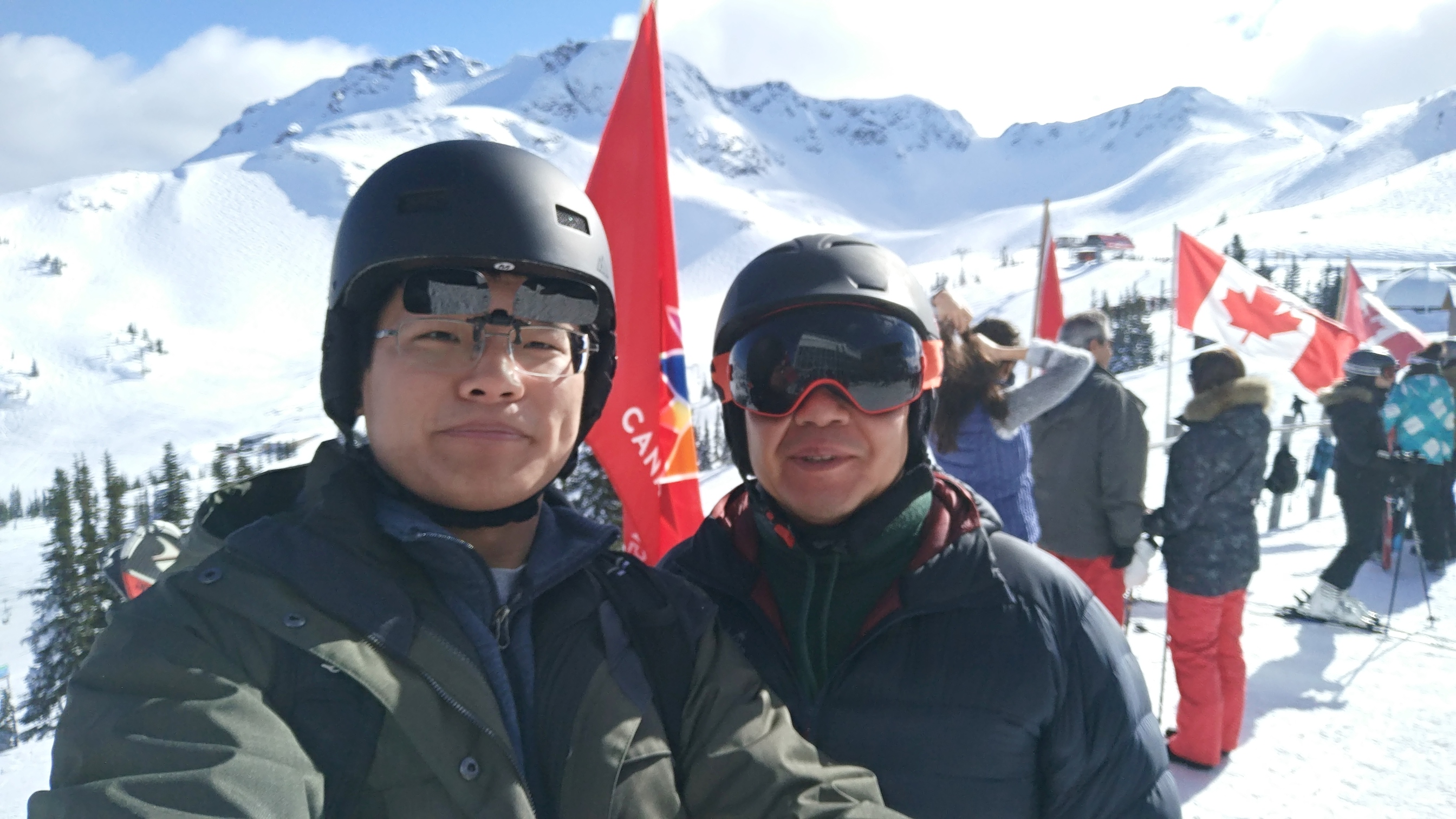
Chun: Yes, that sums it up perfectly. The key point is the contrast in the particular region. With current approaches, the lumen has to be brighter than the background. However, applying the technique to images with black-blood contrast is a possibility.
MRMH: So, what’s next?
Li: We would like to extend the approach to other body parts and other imaging modalities. For now, the tool is built in C++ for Windows, and we are thinking of extending it to other platforms so that it might be adopted more widely. Using cloud computing as well as crowdsourcing may also help in this sense. Another direction is applying iCafe on clinical studies. Here in Paris I am presenting an abstract describing how we used this tool to analyze 163 elderly subjects and found decreased number of branches and increased tortuosity through aging. This is a direction that also looks very promising.
Chun: Li has correctly outlined the future technical developments. Our idea is to keep on generating a quantitative set of parameters that can describe the vasculature at a given time. The resulting data can then be used to shed light on the blood flow conditions, how they interact in brain function, as well as in other tissues, and how they evolve in different diseases and aging processes. Meanwhile, we are planning to build a network of iCafe users with diverse backgrounds and expertise for different research and clinical applications. The feedback from those users will be valuable for iCafe development in the future.