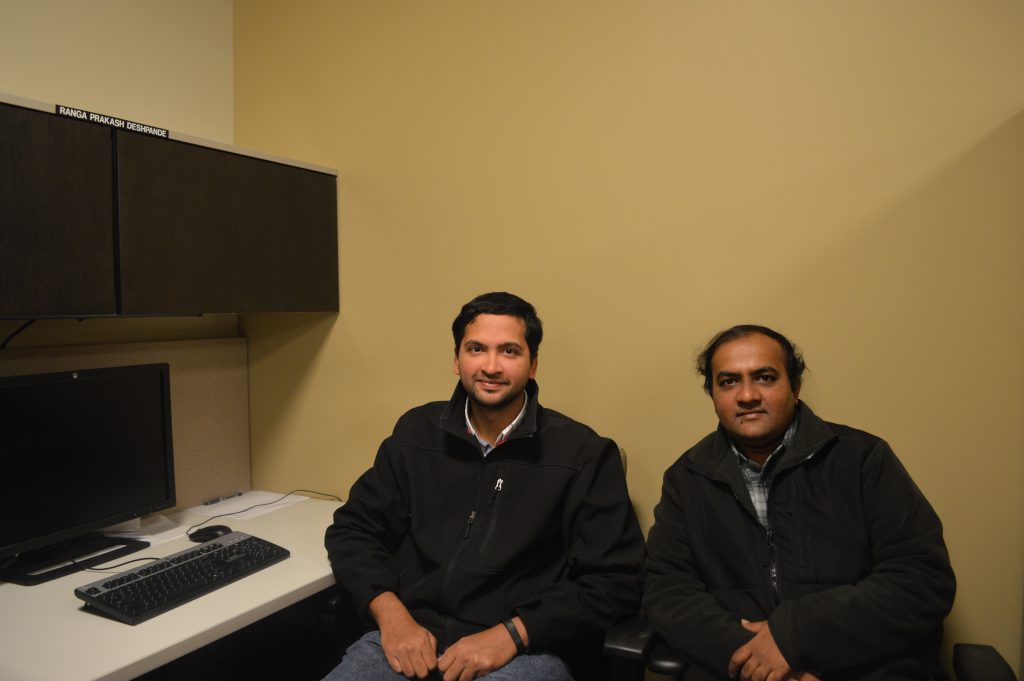
By Tommy Boshkovski
For our second October Editor’s Pick, we were pleased to talk with Dr. Gopikrishna Deshpande and Dr. Ranga Deshpande about their study on the effects of hemodynamic response function (HRF) variability on resting-state fMRI functional connectivity.
MRMH: Can you tell us a bit about yourselves and your background?
Ranga: I did my undergrad studies in electrical engineering in India and my master’s degree in biomedical engineering at the Indian Institute of Science in Bangalore, where I worked on EEG signal processing. I then did a PhD in the MRI Center at Auburn University, where I worked on fMRI connectivity modeling in PTSD. Currently, I am a postdoc at UCLA working on brain imaging of body image and eating disorders.
Gopi: Like Ranga, I am also an alumnus of the Indian Institute of Science in Bangalore. I did my PhD and postdoc in biomedical engineering at Georgia Tech under the supervision of Dr. Xiaoping Hu. Then I came to Auburn University, where I’m an associate professor at the MRI Research Center in the Department of Electrical and Computer Engineering. My research has since been connected to various aspects of fMRI analysis, including the current topic of HRF variability.
MRMH: Can you briefly summarize your paper?
Ranga: fMRI is an indirect measure of brain activity and the HRF is the intermediary between neural activity and its related fMRI BOLD signal. Several years ago it was shown that the HRF is variable across the brain and individuals. Even though it is evident, in principle, that HRF variability will impact functional connectivity estimates from BOLD data, for a long time nothing was done to shed light on its confounding effect on resting-state functional connectivity. This is what prompted us to do this study, in which we looked at the impact of HRF variability on resting-state fMRI functional connectivity in healthy controls.
Gopi: Specifically, what we found is that HRF variability induces false connectivities. Generally, we found more false positives with a mean error of about 15% in connectivity values, and clearly if you’re interpreting specific connections with such large HRF variability then you’re in trouble. We’re not saying that the method we use to deconvolve and estimate the HRF is the best there is, but it is definitely a good one and it has been validated. However, regardless of the method used to deconvolve fMRI data, the variability of the HRF between brain regions and across individuals must be accounted for when performing resting-state fMRI analysis and that, basically, is the message of the paper.
MRMH: Why do you think that the impact of HRF variability on resting-state functional connectivity has been largely overlooked?
Ranga: The situation is rather like what was previously seen with head motion, which used to be corrected simply through rigid body transformation. People were basically satisfied with this, and although they still realized, in a qualitative sense, that head motion causes a lot of artifacts in the data, the problem was otherwise ignored until maybe five years ago, when the effects of residual head motion, after rigid body correction, became more widely appreciated. Essentially, we are facing a similar scenario here.
Gopi: Another point is that you need a good blind deconvolution technique in order to estimate the HRF in every voxel. Such techniques have been under development for many years, and it is only recently that we have seen the emergence of valid ones for resting-state fMRI. This is another reason why this issue has been largely overlooked.
Also, people have previously tended to use resting-state fMRI connectivity for more basic applications. But now it is being used in more sophisticated applications, like connectome fingerprinting or in machine learning algorithms to predict disease status. Obviously, in such applications, HRF-induced errors of the magnitude mentioned earlier really matter.
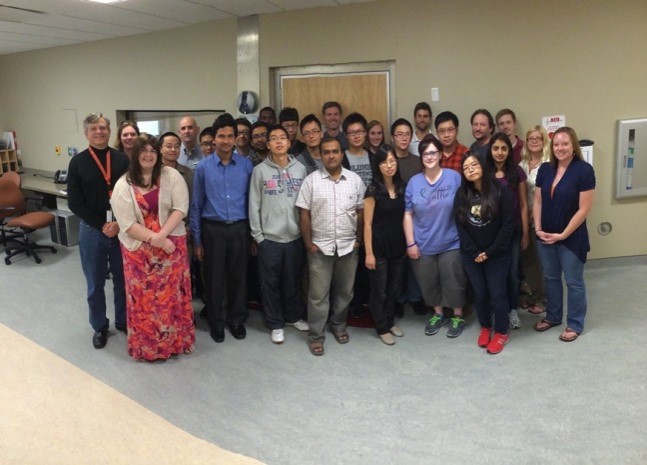
MRMH: You implied that pseudo positive connections have a detrimental impact on fMRI analysis. Can you offer any insight as to why more pseudo positives were detected than pseudo negatives?
Ranga: In our particular setting, pseudo positives are those connections that exist in the original fMRI data, but become weaker in the deconvolved fMRI data. The HRF is quite a smooth function throughout the brain and it is similar in neighboring voxels, which are thus strongly correlated with each other. When we perform deconvolution, this correlation, which is a confounding element, is minimized. On average we observed a reduction in connectivity after deconvolution and that is why we get more pseudo positives.
Gopi: Put another way, there is an HRF-induced correlated component within the connectivity value between two regions, therefore, when its effect is minimized through deconvolution, the average connectivity value is reduced too. That is why we have more pseudo positives than pseudo negatives.
MRMH: In the paper, you stated that the confounds will be even greater on 3T data. Can you clarify this?
Ranga: What was stated is based on theory, not on actual data, and that is what we are looking at right now. And we cannot say by what magnitude the effect will be larger in 3T data, as there is no data allowing us to do so. However, we know there is a theoretical basis for the effect.
Gopi: In high-field fMRI data, it is well known that there is more contribution from small vessels compared to 3T, and the HRF variability is lower for smaller than larger vessels. Because of that weighting towards smaller vessels, we just hypothesized that the HRF variability should be smaller at 7T. However, nobody has really empirically tested whether the HRF variability is larger at 3T, so hopefully, we will show that in our ongoing study.
MRMH: We noticed that you share the same last name, are you related?
Gopi: Ranga is my younger brother.
MRMH: So, what led two brothers to work together?
Gopi: I’ll let Ranga tell the story.
Ranga: [laughs] During my Master’s I was working on EEG in epilepsy and I used to have frequent discussions with Gopi about my ideas on the brain and how I should go forward. That’s how I was drawn to brain imaging and fMRI, and I was particularly interested in the work that Gopi and Tom Denney were doing at Auburn with 7T scanners. In short, I was attracted by both the place and the research. Actually, it was a nice coincidence.
Gopi: Our father is also a scientist and we always used to talk about science and research. I think that probably just rubbed off on Ranga and inspired him to go the same way, I guess.