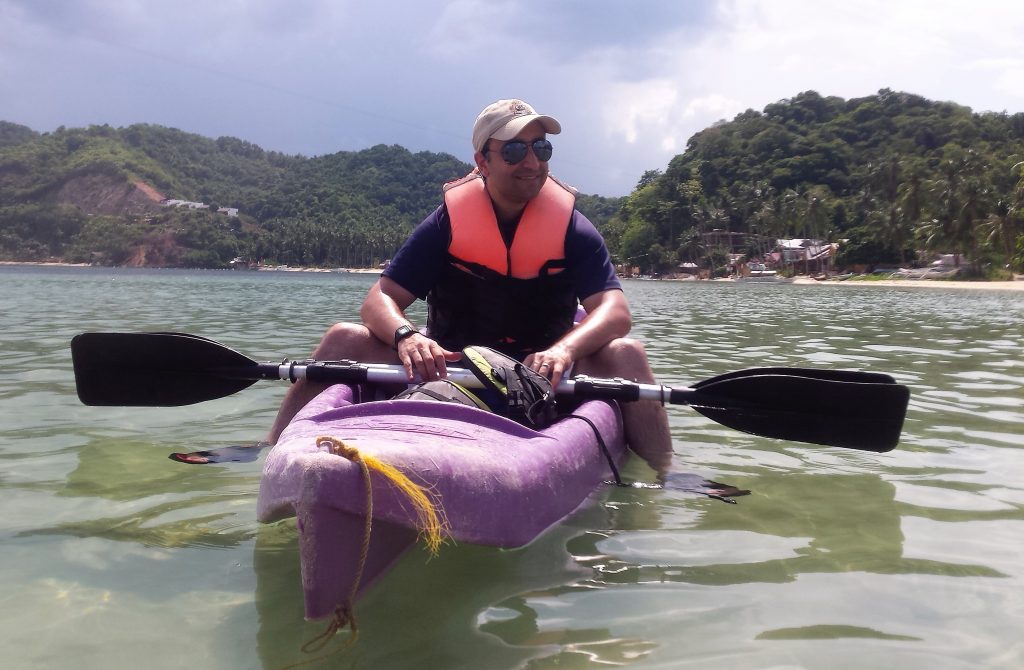
By Mathieu Boudreau
Our first December 2018 Editor’s Pick is from Ouri Cohen and Christian Farrar, researchers at the Martinos Center at the Massachusetts General Hospital (MGH) and Harvard Medical School. Their paper presents an MR fingerprinting approach to quantitative chemical exchange saturation transfer (CEST) imaging. We recently spoke with Ouri and Christian about their project.
MRMH: First of all, we’d like to know a little about you both and your backgrounds.
Ouri: After doing degrees in electrical engineering and physics, I did a PhD in biomedical engineering at Columbia University. I joined the Martinos Center for my postdoc, and this is where Chris and I met. I recently accepted a faculty position at the Memorial Sloan-Kettering Cancer Center in New York City.
Christian: I was a physics undergrad major at the University of Wisconsin, and then came to Boston for grad school at Harvard, where I got my PhD in chemistry. I did a postdoc at MIT, doing high-field dynamic nuclear polarization and electron paramagnetic resonance. I did a second postdoc at MGH doing MRI, and now I’m an assistant professor in the Department of Radiology here at Harvard Medical School and MGH.
MRMH: Could you explain, in simple terms, the concepts of MR fingerprinting and CEST?
Ouri: Everyone knows that a regular fingerprint left at a crime scene can give you a lot of information about a suspect, providing the fingerprint is in your pre-compiled database. Well, the idea behind MR fingerprinting is essentially the same. Basically, by exciting the magnetization with varying acquisition parameters, you can induce signal evolutions that are unique for each tissue type; then, by matching the result to a pre-computed database, you can infer information about the underlying tissue.
Christian: And with CEST, you’re basically measuring contrasts that depend on the chemical exchange rate. By saturating exchangeable protons that exchange with bulk water, you observe a decrease in your water signal. The contrast is going to depend on the exchange rate, and the proton volume fraction of these exchangeable protons. The exchange rate is very sensitive to pH, while the volume fraction gives you information about protein and metabolite concentrations.
MRMH: Could you give us a brief overview of your paper?
Ouri: Traditional CEST pulse sequences are mostly qualitative and have very long scan times. This is the challenge addressed by the paper. Our solution was to integrate an MR fingerprinting paradigm into a CEST acquisition. Unlike traditional MR fingerprinting, where you only vary the flip angle and TR, here the idea was to vary the off-resonance CEST saturation power as well, so as to sensitize the sequence to different exchange rates and concentrations. We found we could reduce the scan time very significantly, to approximately two minutes, as opposed to the 10 minutes or more needed for a traditional CEST sequence. More importantly, we were able to get quantitative CEST maps far more easily than you can when using conventional techniques.
Christian: I’ve been pretty surprised at how well our technique works. In this paper, we only show data from a normal rat, but we now have data from rat stroke models and mouse brain tumor models too. In the brain tumor models you see a decreased proton volume fraction because you’ve got a lot of edema diluting your protein concentrations. And in stroke lesions you see decreased exchange rates consistent with decreased pH, and also slightly decreased proton volume fractions. It’s great that this technique allows you to get a deeper insight into what’s going on in these disease pathologies.
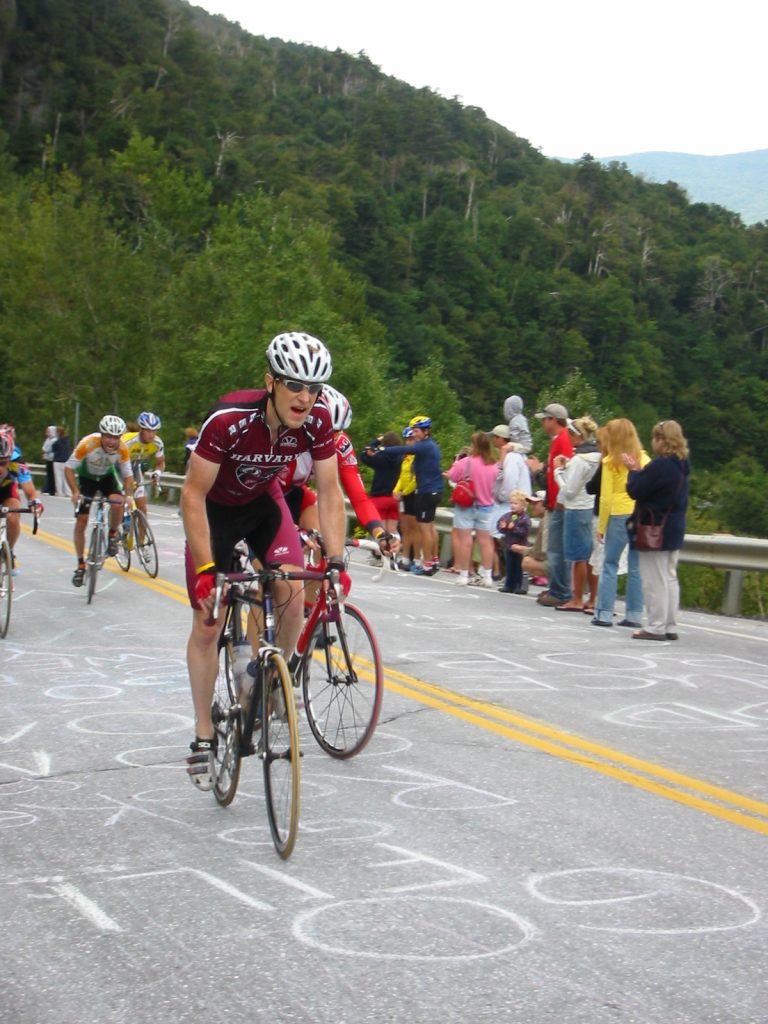
MRMH: How could this technique be further improved in the future?
Ouri: There’s a lot we plan on doing to improve it – I feel like we’ve barely scratched the surface. Just to give an example, the acquisition schedule that we are currently using was selected at random, so it’s not going to be optimal. Optimizing the acquisition schedule will almost certainly improve the discrimination between different tissue types, or further reduce the scan time, so that’s something that we are definitely going to pursue.
Christian: We also want to get whole-brain coverage by exploiting simultaneous multi-slice (SMS) EPI methods, and exciting multiple slices after each saturation pulse while permuting the order of the slices for the different saturation powers; this might also help us to optimize the tissue discrimination even further.
Ouri: From a clinical perspective, I think all these developments would be very important. Essentially, our work is leading up to having a kind of push-key clinical sequence that people can use in different pathologies to generate these useful maps in a reasonable amount of time.
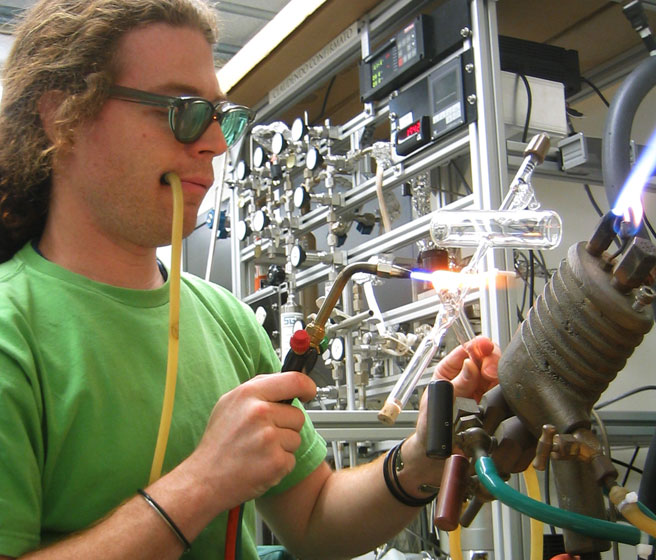
MRMH: What advice would you give to a new grad student wanting to embark on a similar MR fingerprinting project?
Ouri: I would suggest running lots of simulations. The nice thing about MR fingerprinting is that you can simulate a lot of this stuff before even sitting down at the scanner. If you generate ideas and test them in simulations, you can achieve a very quick development cycle, and hopefully get something really interesting going. So I would say, focus on seeing if your work makes sense in simulations, and keep going until you get to something that’s worth trying on the scanner.
Christian: I agree with Ouri that it is very important to run lots of simulations to understand how good your particular acquisition schedule is at discriminating different tissue parameters. I’ve recently noticed that a lot of people trying to quantify chemical exchange rates are getting values that are all over the place. I think this is largely due to the fact that they don’t realize that their fits are not unique. One nice thing about the MR fingerprinting dictionary is that by taking the dot product of the dictionary with itself, you can look at the correlation of the signal trajectories between different tissue parameters and get a sense of how good a particular acquisition schedule is at discriminating between different parameters.